The Future of AI: From One-Size-Fits-All to Personal Digital Partners
Why the next wave of AI will be about amplifying individual excellence, not averaging out capability
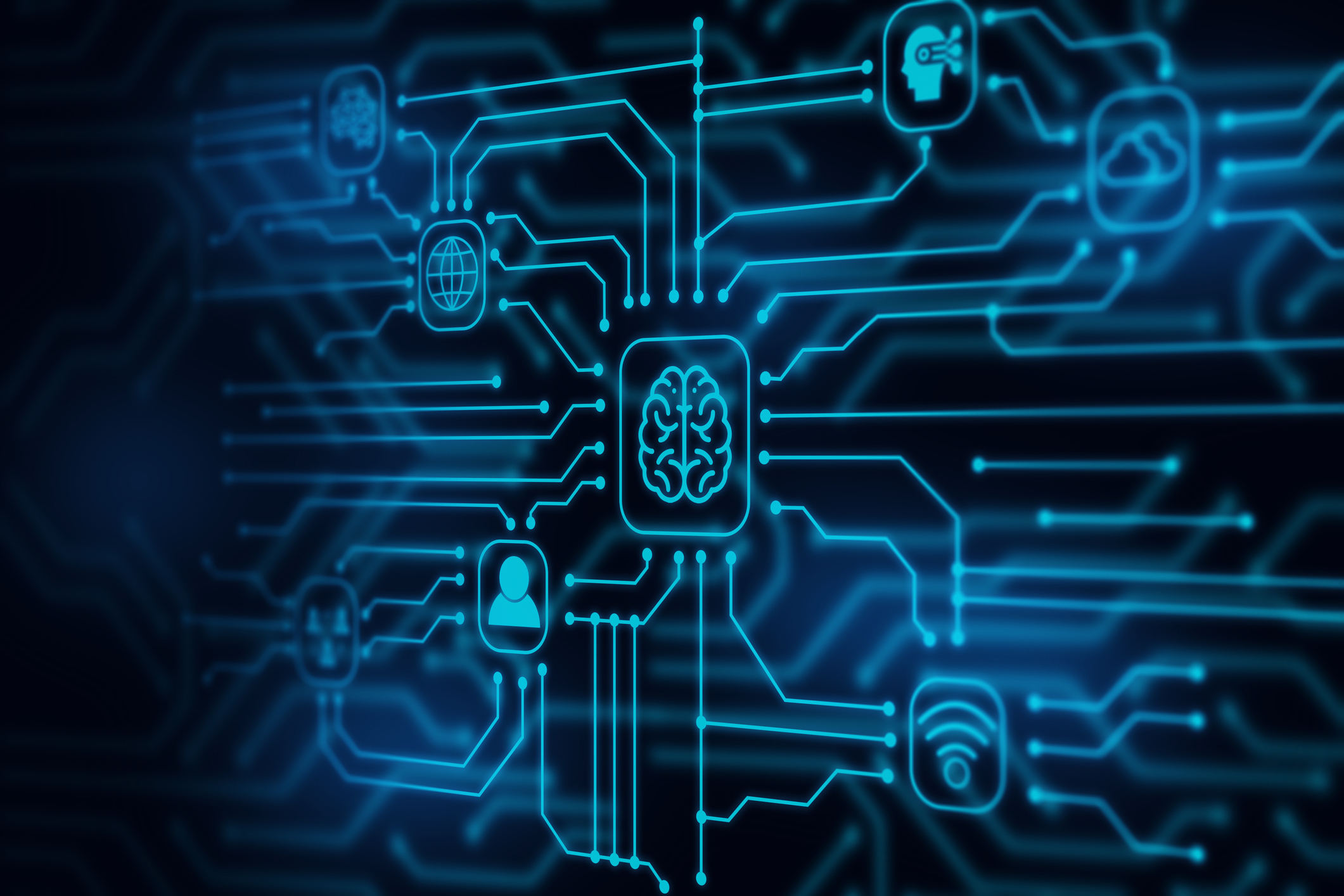
The next time you interact with an AI assistant, notice something peculiar: whether you’re a lawyer drafting contracts, a programmer debugging code, or a writer crafting narratives, you’re essentially talking to the same digital mind. It’s rather like everyone getting their morning coffee from a vending machine – functional, but hardly personalized. This standardization might have served us well in AI’s infancy, but as we stand at the threshold of the next wave of AI evolution, it’s becoming clear that this one-size-fits-all approach is holding us back.
The Limitations of Digital Uniformity
Current AI language models are remarkable generalists, trained on vast oceans of data to be adequately good at almost everything – and therein lies both their strength and their fundamental weakness. Consider for a moment how we approach talent in the human world: When building high-performing teams, we don’t seek out individuals who are moderately capable at everything. Instead, as venture capitalist Ben Horowitz astutely notes in “The Hard Thing About Hard Things,” we hire for strengths rather than an absence of weaknesses. Yet our approach to AI development has paradoxically focused on eliminating weaknesses rather than cultivating exceptional capabilities. The result? AI systems that consistently deliver acceptable but rarely exceptional results. They’re like skilled bureaucrats: competent at following established patterns but lacking the distinctive expertise that defines true mastery in any field.
The Promise of Personalization
The image generation community has already glimpsed a more promising future. Through the development of “Loras” – specialized training modules that can be plugged into base models – they’ve created a framework where just a handful of images can teach an AI to replicate specific artistic styles or characteristics. This elegant solution allows for remarkable customization while maintaining the robust capabilities of the underlying model. Text-based AI hasn’t yet achieved this level of adaptability. Current systems require users to repeatedly provide context documents, with no real learning or adaptation taking place. Imagine having to explain your preferences to your coffee barista every single morning – it’s inefficient and fundamentally limits the depth of customization possible.
The Path Forward
The future of AI lies not in building bigger, more generalized models, but in developing systems that can adapt and specialize. Picture an AI that doesn’t just process your emails but understands your communication style, professional context, and personal priorities. One that codes not just functionally, but in a way that aligns with your team’s architectural patterns and coding philosophy.
This transformation will require a shift in how we think about AI capabilities. Instead of asking “What can this AI do?” we should be asking “What unique value do I bring, and how can AI amplify that?” For a writer, this might mean an AI that understands not just grammar and structure, but your distinct voice and narrative preferences. For a programmer, it could mean an AI that grasps your architectural patterns and coding idioms, becoming a more effective collaborator rather than just a sophisticated autocomplete tool.
The business implications are profound. Organizations will need to start thinking about their AI strategy not in terms of which existing tools to adopt, but rather how to capture and amplify their unique institutional knowledge and capabilities through AI. Success in this new paradigm will depend on our ability to clearly articulate what makes us exceptional – both as individuals and organizations – and to effectively transfer that knowledge to our AI systems.
As we stand at this crossroads, it’s worth remembering that the goal isn’t to replace human expertise but to amplify it. The future belongs not to those who can best utilize generic AI tools, but to those who can effectively teach AI systems to complement and enhance their unique strengths.
The challenge ahead isn’t technological – it’s conceptual. We need to shift our thinking from AI as a universal tool to AI as a personalized partner, capable of learning and adapting to our individual needs and strengths. Only then will we unlock the true potential of this technology, moving beyond the current paradigm of acceptable mediocrity to one of exceptional capability.